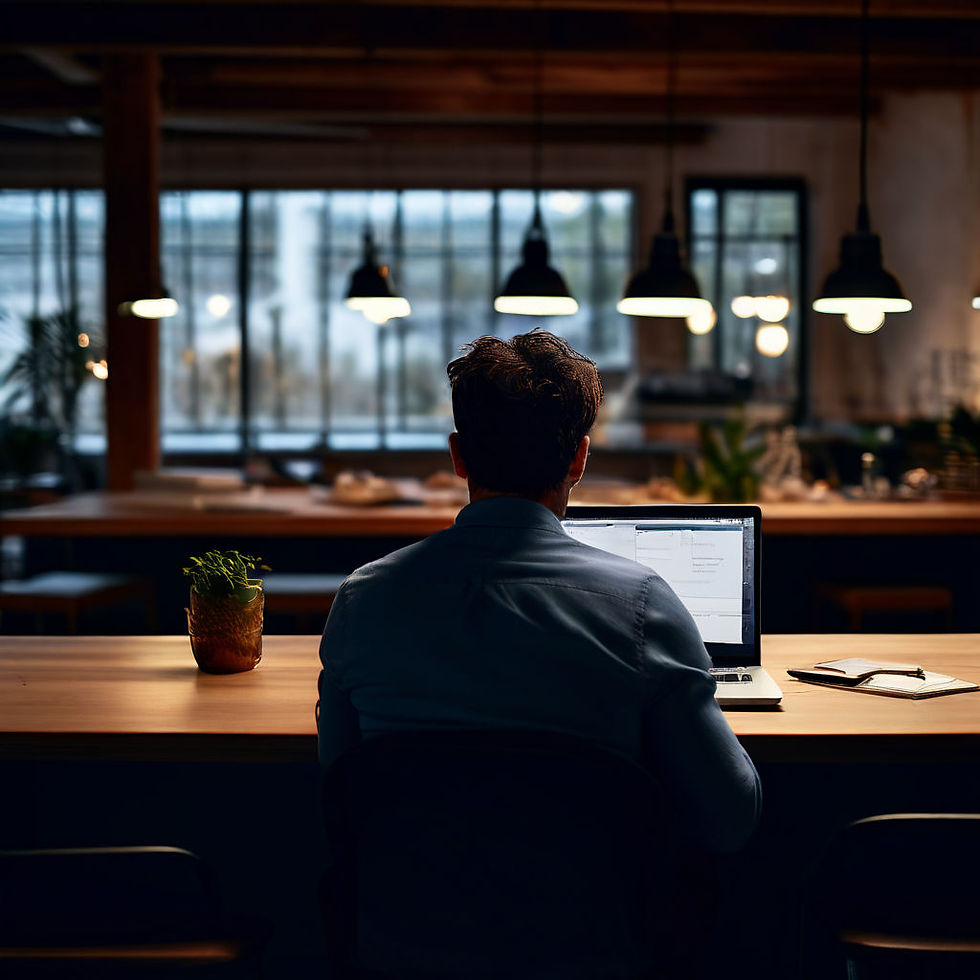
In today’s rapidly evolving business landscape, the ability to forecast demand accurately has become a pivotal element in maintaining a competitive edge. AI-driven demand forecasting emerges as a transformative power, providing businesses with the tools necessary to navigate market fluctuations with agility and precision. This sophisticated approach transcends traditional forecasting methods, offering a nuanced understanding of consumer behavior, identifying patterns amidst chaos, and helping organizations prepare for the unpredictable nature of their respective markets.
At its core, AI-driven demand forecasting utilizes machine learning algorithms and advanced analytics to process vast data sets, uncover trends, and generate predictions that were previously unattainable. As professionals across sectors delve into the intricacies of this technology, it becomes crucial to explore its applications, benefits, complexities, and future implications in a thorough and structured manner.
Understanding the Fundamentals of AI-Driven Demand Forecasting
AI-driven demand forecasting is not merely a technical advancement; it represents a paradigm shift in how businesses comprehend and anticipate market needs. By leveraging data sourced from various internal and external channels — ranging from historical sales and customer behavior data to current economic indicators — organizations can create increasingly accurate demand forecasts.
The Role of Data in AI Forecasting
Data is the lifeblood of AI systems, and demand forecasting is no exception. The effectiveness of AI-driven forecasting hinges on the quality, quantity, and relevance of data.
Organizations often gather data from diverse sources, including:
- Point of Sale (POS) systems: Capturing real-time sales data to monitor current trends.
- Market Research: Integrating external market studies to enrich insights.
- Social Media Analytics: Understanding consumer sentiment, brand perception, and upcoming trends through social listening.
- Economic Indicators: Considering macroeconomic factors such as unemployment rates, GDP growth, and industry-specific drivers.
This deluge of data, when deftly analyzed, enables businesses to not only predict demand with higher accuracy but also identify underlying factors influencing customer behavior.
Machine Learning Models and Algorithms
The intricacy of AI-driven demand forecasting lies largely in the choice of machine learning models. Different algorithms — ranging from time series analysis and regression models to neural networks — serve varying purposes within forecasting frameworks. For instance, neural networks can decipher intricate patterns in large datasets, making them ideal for environments with non-linear relationships, while simpler regression models may suffice for linear correlations.
A noteworthy case is Instacart, which utilizes machine learning to forecast the demand for grocery items across different regions. By leveraging its vast database of user behavior, sales transactions, and seasonality, Instacart can efficiently predict which items will be in demand, thus optimizing inventory levels and reducing waste.
Benefits of AI-Driven Demand Forecasting in Navigating Market Fluctuations
The potential advantages of implementing AI for demand forecasting are multifaceted. While traditional forecasting methods often rely on historical data alone, AI supplements this perspective with predictive insights that can proactively prepare organizations for fluctuations.
Enhanced Accuracy and Responsiveness
An AI-driven approach enables companies to achieve remarkable improvements in forecasting accuracy — sometimes exceeding traditional methods by over 20 percent. This leap allows for heightened responsiveness to changing market dynamics. For example, Coca-Cola employs AI algorithms to analyze consumption patterns and anticipate demand, which in turn facilitates optimized production and distribution strategies.
Cost Efficiency
Accurate demand forecasting directly contributes to cost reduction in various areas, such as inventory management and supply chain operations. By minimizing overstock and stockouts through precise predictions, organizations can significantly lower holding costs and waste. Zara, the global fashion retailer, exemplifies this approach as it adjusts production schedules based on AI-driven forecasts to keep inventory lean and relevant — leading to substantial savings and increased profitability.
Sustainability and Waste Reduction
As companies face increasing pressure to demonstrate sustainability — and consumers seek eco-conscious brands — AI-driven demand forecasting plays a vital role in minimizing waste. Accurate demand predictions facilitate better alignment of supply and demand, reducing surplus production that contributes to environmental strain.
Complexities and Challenges in Implementing AI-Driven Forecasting
While the prospects of AI-driven demand forecasting are exciting, professionals must also navigate several challenges that can hinder successful implementation. These complexities require a thoughtful approach and strategic management to harness the capabilities of AI effectively.
Data Privacy and Quality Concerns
Data privacy regulations, such as GDPR, add layers of complexity to the responsible use of personal data for demand forecasting. Organizations must ensure compliance while balancing the need for comprehensive data sets that drive accurate forecasts. Moreover, compromised data quality — stemming from integration across disparate sources — can lead to flawed predictions, ultimately affecting decision-making processes.
Integration of Traditional and AI Methods
Many organizations have established demand forecasting processes that predate the advent of AI technologies. Transitioning to an AI-driven model necessitates not just new systems but also a cultural shift within the organization. There may be resistance from employees accustomed to traditional methodologies, which can undermine the effectiveness of AI implementation.
For illustrative purposes, Procter & Gamble faced significant challenges while integrating AI into its forecasting systems. Initially, the company relied heavily on conventional methods, which posed difficulties when trying to implement machine learning. By investing in comprehensive training programs and engaging employees in the transition process, P&G successfully bridged the gap, resulting in a 50% reduction in forecast error.
Future Implications and Trends in AI-Driven Demand Forecasting
Looking ahead, several trends are poised to shape the future of AI-driven demand forecasting, influencing how companies prepare for market fluctuations.
The Rise of Autonomous Forecasting Systems
As AI technologies continue to evolve, the advent of autonomous forecasting systems — self-learning algorithms capable of continually improving their forecasting models — will likely emerge. These systems can adapt to new data inputs in real-time, enhancing accuracy and providing businesses with near-instantaneous insights for decision-making processes.
Cross-Industry Applications and Collaboration
With the growing applications of AI in data-driven solutions, cross-industry collaborations will become increasingly vital. Companies can share data insights (while adhering to privacy laws) to create more comprehensive forecasting models. For instance, partnerships between food and beverage manufacturers and retailers could enhance demand predictions during seasonal peaks, benefiting both parties.
Ethical Considerations and Responsible AI
As AI becomes a more integral part of demand forecasting, ethical considerations will increasingly come to the forefront. Companies must not only ensure transparency in their AI processes but also develop strategies that mitigate bias and promote equitable access to AI-driven insights.
Conclusion
AI-driven demand forecasting represents a frontier in data analytics that can profoundly transform how organizations anticipate and respond to market fluctuations. By harnessing the power of advanced algorithms and comprehensive datasets, businesses can unlock unprecedented levels of accuracy and agility. However, the complexities of implementation and future implications also necessitate careful consideration — from data privacy to ethical challenges.
As industries continue to evolve amidst rapid technological advancements, those who invest in understanding and adopting AI-driven forecasting will position themselves favorably in the marketplace. Building a robust framework for demand forecasting not only enables businesses to respond to consumer needs with precision but also prepares them to thrive amid uncertainty.
Thank you for reading!
For more in-depth insights on innovative solutions in your industry and to see how AutomateLogix can help transform your operations, visit us at www.automatelogix.com.
Stay ahead with cutting-edge solutions tailored to your needs.
Comments